Cybersecurity Defenders Are Expanding Their AI Toolbox
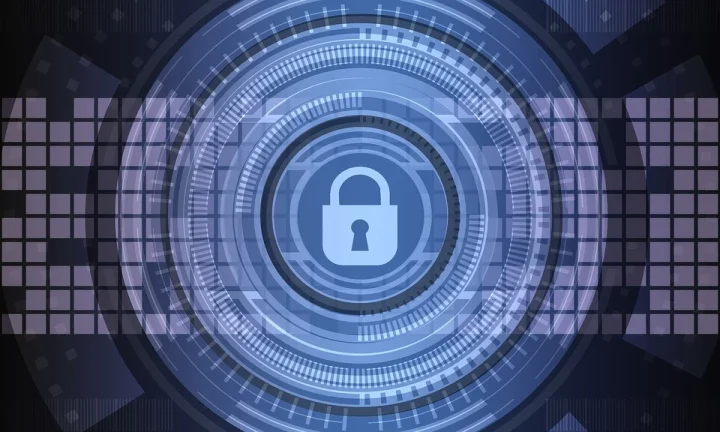
[ad_1]
Cybersecurity scientists have taken a crucial phase towards harnessing a sort of artificial intelligence recognised as deep reinforcement discovering, or DRL, to protect pc networks.
Cybersecurity – artistic impact. Impression credit history: Pixabay (Cost-free Pixabay license)
When faced with sophisticated cyberattacks in a arduous simulation environment, deep reinforcement learning was productive at stopping adversaries from achieving their plans up to 95 percent of the time. The end result gives promise for a job for autonomous AI in proactive cyber protection.
Researchers from the Office of Energy’s Pacific Northwest National Laboratory documented their results in a investigation paper and presented their get the job done Feb. 14 at a workshop on AI for Cybersecurity all through the annual assembly of the Affiliation for the Improvement of Synthetic Intelligence in Washington, D.C.
The beginning stage was the improvement of a simulation setting to exam multistage assault eventualities involving distinctive varieties of adversaries. Development of this kind of a dynamic attack-protection simulation setting for experimentation itself is a earn. The ecosystem delivers researchers a way to examine the success of distinct AI-dependent defensive techniques less than controlled take a look at options.
This kind of equipment are essential for evaluating the performance of deep reinforcement mastering algorithms. The process is rising as a powerful conclusion-support tool for cybersecurity experts—a defense agent with the ability to understand, adapt to promptly changing situation, and make selections autonomously.
Though other types of synthetic intelligence are common to detect intrusions or filter spam messages, deep reinforcement discovering expands defenders’ talents to orchestrate sequential decision-earning options in their every day face-off with adversaries.
Deep reinforcement studying provides smarter cybersecurity, the means to detect adjustments in the cyber landscape before, and the option to acquire preemptive ways to scuttle a cyberattack.
DRL: Conclusions in a broad assault space
“An efficient AI agent for cybersecurity wants to feeling, understand, act and adapt, based on the information and facts it can assemble and on the effects of selections that it enacts,” said Samrat Chatterjee, a details scientist who offered the team’s work. “Deep reinforcement discovering retains excellent likely in this space, in which the number of process states and action alternatives can be large.”
DRL, which combines reinforcement studying and deep finding out, is in particular adept in predicaments exactly where a collection of choices in a complex surroundings need to have to be manufactured. Excellent conclusions leading to appealing outcomes are strengthened with a good reward (expressed as a numeric price) lousy options major to unwanted outcomes are discouraged via a damaging expense.
It is similar to how men and women understand many responsibilities. A baby who does their chores may well acquire positive reinforcement with a preferred playdate a little one who doesn’t do their function gets destructive reinforcement, like the takeaway of a electronic device.
“It’s the exact same notion in reinforcement mastering,” Chatterjee explained. “The agent can pick out from a established of actions. With each and every action comes responses, great or lousy, that gets part of its memory. There’s an interaction among exploring new alternatives and exploiting earlier experiences. The aim is to develop an agent that learns to make great conclusions.”
Open AI Health club and MITRE ATT&CK
The group employed an open-resource computer software toolkit acknowledged as Open up AI Health and fitness center as a basis to produce a personalized and managed simulation ecosystem to consider the strengths and weaknesses of 4 deep reinforcement studying algorithms.
The team made use of the MITRE ATT&CK framework, produced by MITRE Corp., and incorporated 7 ways and 15 approaches deployed by three distinct adversaries. Defenders were geared up with 23 mitigation actions to try out to halt or stop the progression of an assault.
Stages of the assault included methods of reconnaissance, execution, persistence, protection evasion, command and handle, collection and exfiltration (when facts is transferred out of the program). An assault was recorded as a get for the adversary if they efficiently arrived at the final exfiltration stage.
“Our algorithms run in a competitive environment—a contest with an adversary intent on breaching the procedure,” said Chatterjee. “It’s a multistage attack, the place the adversary can pursue numerous assault paths that can change over time as they test to go from reconnaissance to exploitation. Our challenge is to show how defenses dependent on deep reinforcement mastering can halt this kind of an attack.”
DQN outpaces other methods
The workforce educated defensive agents dependent on 4 deep reinforcement studying algorithms: DQN (Deep Q-Community) and three variations of what is known as the actor-critic solution. The agents have been properly trained with simulated information about cyberattacks, then tested against assaults that they had not observed in coaching.
DQN carried out the greatest.
- The very least subtle assaults (centered on different degrees of adversary ability and persistence): DQN stopped 79 p.c of assaults midway via assault phases and 93 % by the closing phase.
- Reasonably refined attacks: DQN stopped 82 per cent of attacks midway and 95 per cent by the last stage.
- Most complex assaults: DQN stopped 57 p.c of assaults midway and 84 percent by the remaining stage—far increased than the other 3 algorithms.
“Our intention is to develop an autonomous defense agent that can learn the most likely upcoming move of an adversary, system for it, and then respond in the very best way to guard the method,” Chatterjee claimed.
Irrespective of the progress, no 1 is all set to entrust cyber protection entirely up to an AI technique. In its place, a DRL-centered cybersecurity procedure would require to perform in concert with human beings, said coauthor Arnab Bhattacharya, previously of PNNL.
“AI can be very good at defending versus a certain strategy but isn’t as superior at understanding all the techniques an adversary could choose,” Bhattacharya claimed. “We are nowhere near the stage where AI can swap human cyber analysts. Human opinions and assistance are important.”
In addition to Chatterjee and Bhattacharya, authors of the AAAI workshop paper involve Mahantesh Halappanavar of PNNL and Ashutosh Dutta, a former PNNL scientist. The operate was funded by DOE’s Place of work of Science. Some of the early get the job done that spurred this particular study was funded by PNNL’s Mathematics for Artificial Reasoning in Science initiative via the Laboratory Directed Exploration and Improvement plan.
Published by Tom Rickey
[ad_2]
Resource backlink As the use of artificial intelligence (AI) continues to expand, cybercrime is evolvingrapidly to keep pace—which is why it’s becoming more important than ever for cybersecurity defenders to expand their AI toolbox.
In recent months, investmen t in AI-powered cyber defense has skyrocketed. According to the Boston Consulting Group, 59% of security executives surveyed expect to introduce AI across their operations within the next three years. Recently, one AI-based startup, Cylance, was acquired by BlackBerry for $1.4 billion in cash. This is expected to jumpstart the rush to adopt AI-driven approaches to cybersecurity.
AI has also proven to be a powerful match for rapidly advancing cyber threats. AI can be used to automate tasks, improve detection and categorization of threats, and analyze the security posture of an organization. In addition, AI can generate customized responses to attacks before they’re even detected. These capabilities give cybersecurity defenders the opportunity to respond more quickly to new threats, improve their detection success rates, and reduce false positives.
One major advantage of AI-driven cyber defense is that it can operate at a significantly greater scale than manual methods, enabling defenders to process huge amounts of data and identify more complex threats. In addition, AI can navigate and coordinate systems that are much more interconnected than manually managed environments, facilitating a faster response to threats.
Some experts predict the future of AI-driven cyber defense could involve AIs autonomously fighting smaller-scale, continuous attacks. For example, AI-driven cybersecurity defenders could detect new attacks and devise countermeasures in real-time, eliminating the need for manual intervention.
As AI-driven cybersecurity continues to evolve, it’s clear that defenders will need to rely more heavily on AI as they face off against increasingly sophisticated attackers. Cybersecurity firms are continuing to invest in AI-driven solutions to combat these threats, and the rising demand for AI-driven cybersecurity capabilities means that defenders must innovate to stay ahead of the curve.