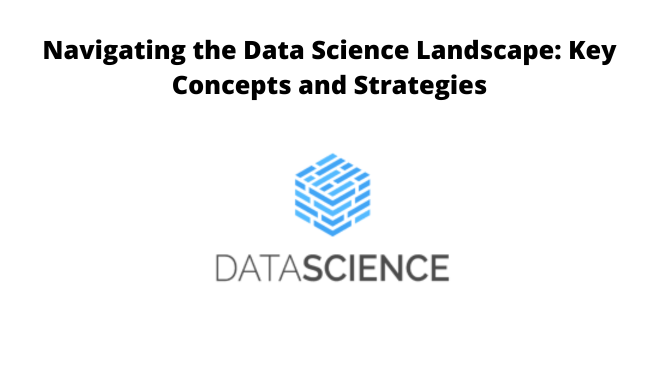
Introduction:
In the dynamic realm of technology, Data Science has emerged as a driving force behind innovation and informed decision-making. As businesses strive to harness the power of data, understanding the key concepts and strategies in Data Science becomes essential.
In this blog post, we’ll delve into fundamental principles and practical strategies that underpin the field, providing a roadmap for both beginners and seasoned professionals. Secure a career in the dominant domain of Data Analytics by joining the Data Science Training in Hyderabad course by Kelly Technologies.
Foundations of Data Science:
-
Data Types and Sources:
- Understanding the distinction between structured and unstructured data.
- Recognizing diverse data sources, including databases, APIs, and streaming data.
-
Descriptive Statistics:
- Grasping the basics of descriptive statistics to summarize and visualize data.
- Exploring measures of central tendency, dispersion, and correlation.
-
Inferential Statistics:
- Unraveling the principles of inferential statistics for drawing conclusions about populations based on samples.
- Exploring hypothesis testing and confidence intervals.
-
Data Visualization:
- Embracing the art of storytelling through data visualization.
- Choosing the right charts and graphs to effectively communicate insights.
Essential Strategies in Data Science:
-
Feature Engineering:
- Understanding the importance of feature selection and transformation.
- Unraveling the impact of feature engineering on model performance.
-
Model Selection and Evaluation:
- Navigating the landscape of machine learning algorithms.
- Employing cross-validation and hyperparameter tuning for robust model evaluation.
-
Explainability and Interpretability:
- Recognizing the significance of explainable AI.
- Implementing techniques to interpret complex models for stakeholders.
-
Data Ethics and Bias Mitigation:
- Addressing ethical considerations in data collection and model development.
- Implementing strategies to mitigate bias and ensure fairness in predictions.
-
Scalability and Big Data:
- Adapting Data Science workflows for scalability.
- Exploring technologies like Apache Spark for handling large datasets.
The Human Element in Data Science:
-
Collaboration and Communication:
- Emphasizing the importance of cross-functional collaboration between data scientists, domain experts, and business stakeholders.
- Communicating findings in a clear and compelling manner.
-
Continuous Learning:
- Embracing a mindset of continuous learning in the ever-evolving field of Data Science.
- Leveraging online courses, conferences, and community engagement.
The Future Landscape:
-
AI and Machine Learning Integration:
- Recognizing the symbiotic relationship between Data Science and artificial intelligence.
- Exploring advancements in machine learning techniques and frameworks.
-
Automated Machine Learning (AutoML):
- Understanding the role of AutoML in streamlining the model development process.
- Evaluating the trade-offs between automation and customization.
Conclusion:
As Data Science continues to shape the technological landscape, mastering its fundamental concepts and adopting effective strategies is paramount. From understanding the basics of data types to navigating the ethical considerations, a holistic approach to Data Science ensures not only technical proficiency but also responsible and impactful use of data. By embracing continuous learning and staying attuned to the evolving landscape, individuals and organizations can unlock the full potential of Data Science and chart a course towards data-driven success.